What's in this article?
Lead scoring is meant to simplify the sales process, and yet doing it well has felt increasingly complicated and out of reach.
We’re here to explain how lead scoring can finally be made manageable, efficient, automated, and accurate in our ultimate guide to lead scoring.
Beyond a basic understanding of what is lead scoring as well as your lead scoring tools and options, we’ll also give you the concrete information you need to implement lead scoring successfully as the options for managing it continue to grow.
Use it to actually increase conversions and boost lead scoring ROI within your industry in a way that best fits the needs of your organization.
What is lead scoring?
Lead scoring is the system that sales and marketing teams use to assign value to each new prospect, which is meant to determine how important the lead, or potential customer, is to the business. By ranking each lead based on various data points, sales and marketing gain clarity on which leads to engage and when, prioritizing leads based on the probability of each one turning into a sale.
The lead scoring definition is changing
Past lead scoring was done by manually setting stagnant rules with weighted lead features and making assumptions about what qualifies a lead. New lead scoring innovations use artificial intelligence and machine learning to more accurately predict outcomes with each lead automatically and in real-time.
Fortunately, new predictive lead scoring optimizes how businesses rank, prioritize and work leads. Rather than basing lead values on best guesses set with rules that easily become stagnant, AI/ML lead scoring now provides predictive values for each lead to offer intelligent decision support. This empowers sales and marketing teams with accuracy that wasn’t previously possible.
Get our free download to learn the top 5 ways to boost sales with AI.
Why you need new lead scoring methods to stay competitive
Pay attention to new lead scoring models. They’re advancing the first step in lead management, taking lead scoring from clunky and static and transforming it into an efficient and revenue-increasing process — all with the use of artificial intelligence and machine learning.
That may be one reason marketing and sales departments prioritize AI technology and machine learning for their success more than any other department (40%).
How predictive lead scoring applies to your business
Whether you buy thousands of leads, invest in marketing to generate them or a combination of both, you’re paying for sales opportunities and receiving thousands of rich data points.
However, if lead data is not evaluated, and instead left static, it’s going to underperform. Manually setting and updating lead scoring rules is difficult to manage accurately as the market, your campaigns and products or services evolve. Either way, you’ll close far fewer sales than expected.
Lead scoring is a concrete way to avoid the pitfalls of managing this data. Thousands of complex data points are combined into one lead scoring model, which provides intelligent decision support to increase conversions.
Data quality issues have challenged businesses for years. Now 48% of companies use data analysis, machine learning, or AI tools to address data quality issues.
Learn more about how high-quality lead scoring impacts business: The Ultimate Guide to Optimizing Lead Scoring and Growing Business.
Why is the shift in lead scoring important now?
CRM and lead management systems driven by static rules and reports, are quickly becoming obsolete and creating challenges for organizations that need improved lead scoring ROI from quality lead management.
New and improved lead scoring models are becoming the norm, allowing businesses to optimize lead scoring with lead management innovations. In 2021, 86% of CEOs said AI is mainstream technology in their office, and this is expected to grow.
Integrated, intelligent and predictive AI lead scoring boosts the performance of each lead and each salesperson.
AI is improving how we manage sales and marketing overall
According to the 2022 McKinsey Global Survey on AI, revenue increases from AI adoption are most often reported in these business functions:
- 70% of respondents reported revenue increases in marketing and sales
- 70% said product and/or service development
- 65% said strategy and corporate finance
For comparison, in 2018, manufacturing and risk were the two functions where the largest set of respondents reported value from using AI.
In addition, optimization of service operations has taken the top spot for AI use cases, with product/service development and marketing and sales following closely behind.
Increase conversions from the start with AI lead assignment. Download our free guide to learn more.
How does lead scoring optimize sales?
Lead scoring is an important part of any good lead management system, helping marketing and sales teams quantify how qualified leads are.
This ideally informs good decisions about how to prioritize, distribute and work each lead to focus on sales-ready leads that will increase conversions.
To optimize sales with lead scoring, lead data needs to be collected, and (here comes the tricky part) it needs to be interpreted accurately to assign value to each lead as they’re generated and as they continue to engage with your organization.
These scores then translate to the next steps, like pushing a lead further down the sales funnel with a new email campaign or distributing the lead to a salesperson to reach them with a phone call or text.
Having leads is only valuable to your business if you can convert many of those leads into customers. Accurate lead scoring is one of the first steps that will optimize sales conversions.
Read on to learn the different ways to manage and optimize lead data and lead scores.
Lead scoring alignment between sales and marketing
One important piece that makes lead scoring successful is setting agreements between the marketing and sales teams within an organization. This includes clearly defining concepts like what makes a lead qualified, how to identify qualified leads and what to do next with those leads.
What is an MQL compared to an SQL?
To help organizations prioritize which leads are most likely to convert, leads are first qualified as a Marketing Qualified Lead and then ideally move on to become a Sales Qualified Lead.
- A Marketing Qualified Lead (MQL) is someone who has shown interest in an organization’s product or service as seen through their engagement with various marketing efforts. The marketing team considers leads based on certain criteria. Once a lead is qualified, it gets passed along to the sales team to engage further.
- A Sales Qualified Lead (SQL) is a lead that is a prospective customer who has shown they’re ready to buy or close a sale. Sales Qualified Leads typically have gone through a few levels of qualification before reaching the sales team and being contacted by a salesperson. They’re likely first deemed Marketing Qualified Leads (MQLs) after various engagements with marketing efforts.
So an SQL vs an MQL can show the difference between how engaged a lead is and how sales-ready they are.
How to qualify leads with lead scoring
Lead scoring is used to help sales and marketing teams determine a value or threshold that would deem a lead qualified.
The challenge is that there are many factors that impact how someone might qualify a lead. To do this well — without bias, assumption, or human error — requires the use of deeper analytics.
Beyond the lead data itself, you also need to have an understanding of the marketing and sales team’s goals, capabilities, and capacity.
When it comes to lead scoring, organizations will have the most success if they support both their marketing and sales teams, allowing them to be aligned in their goals and have access to tools that will set them up for success.
Accurate lead scoring capabilities will ultimately help to measure the overall performance of the sales cycle for customers from start to finish.
Optimize your sales and marketing efforts. Check out our Guide to Lead Management: How to Convert More Leads into Deals.
5 reasons to use a lead scoring model
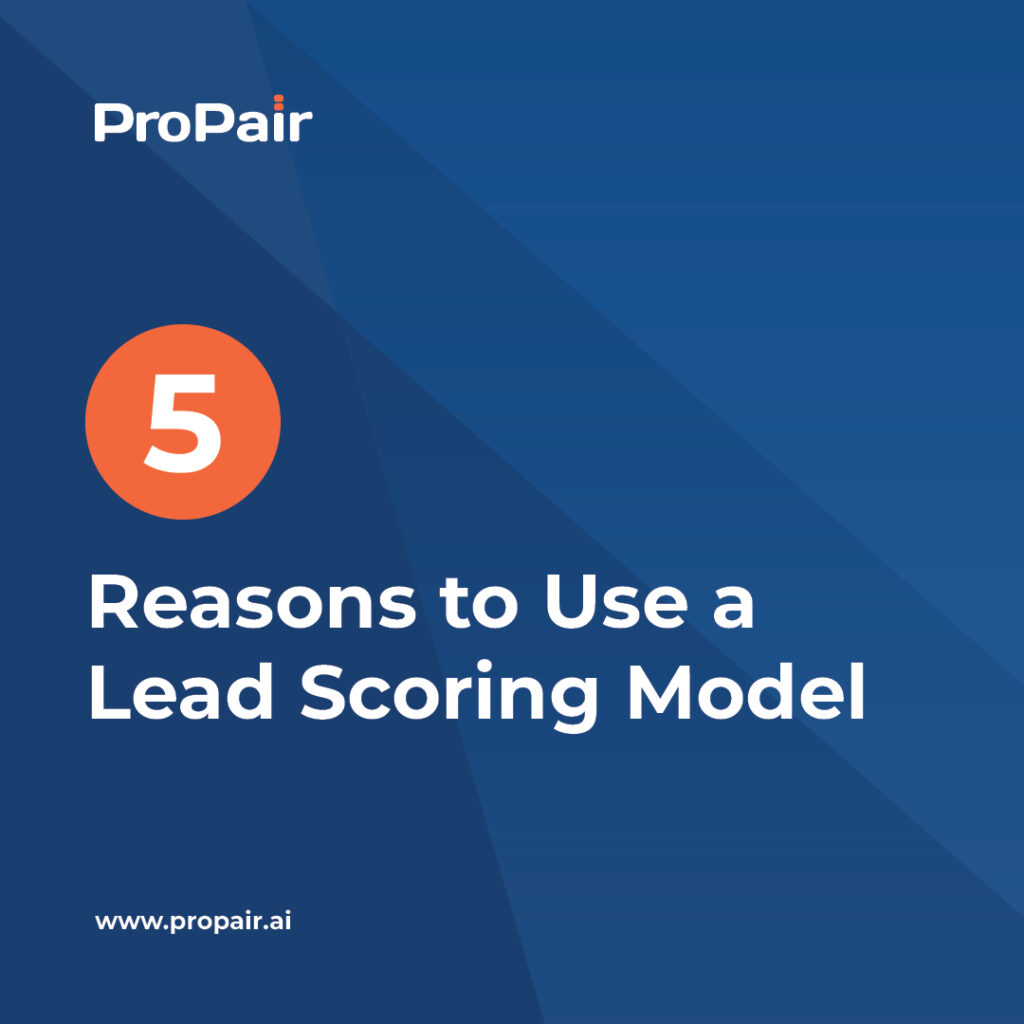
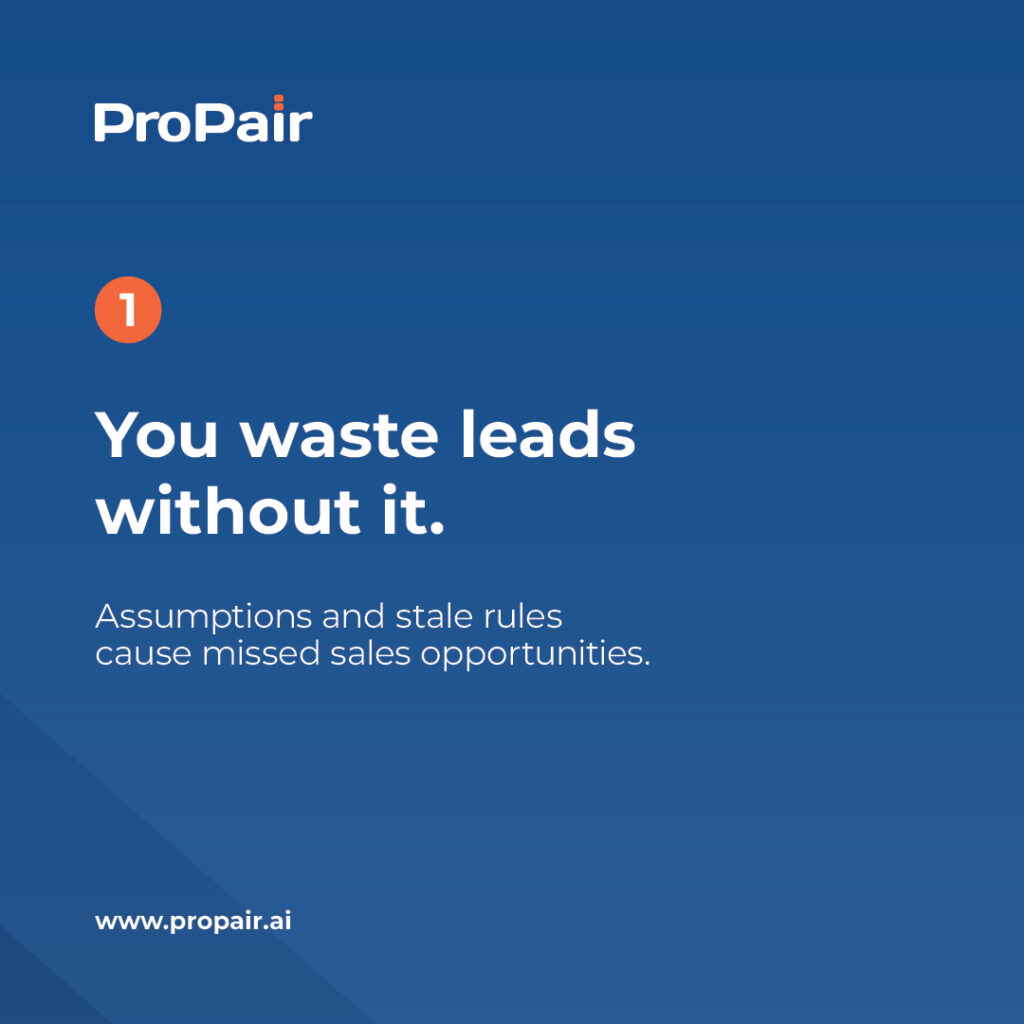
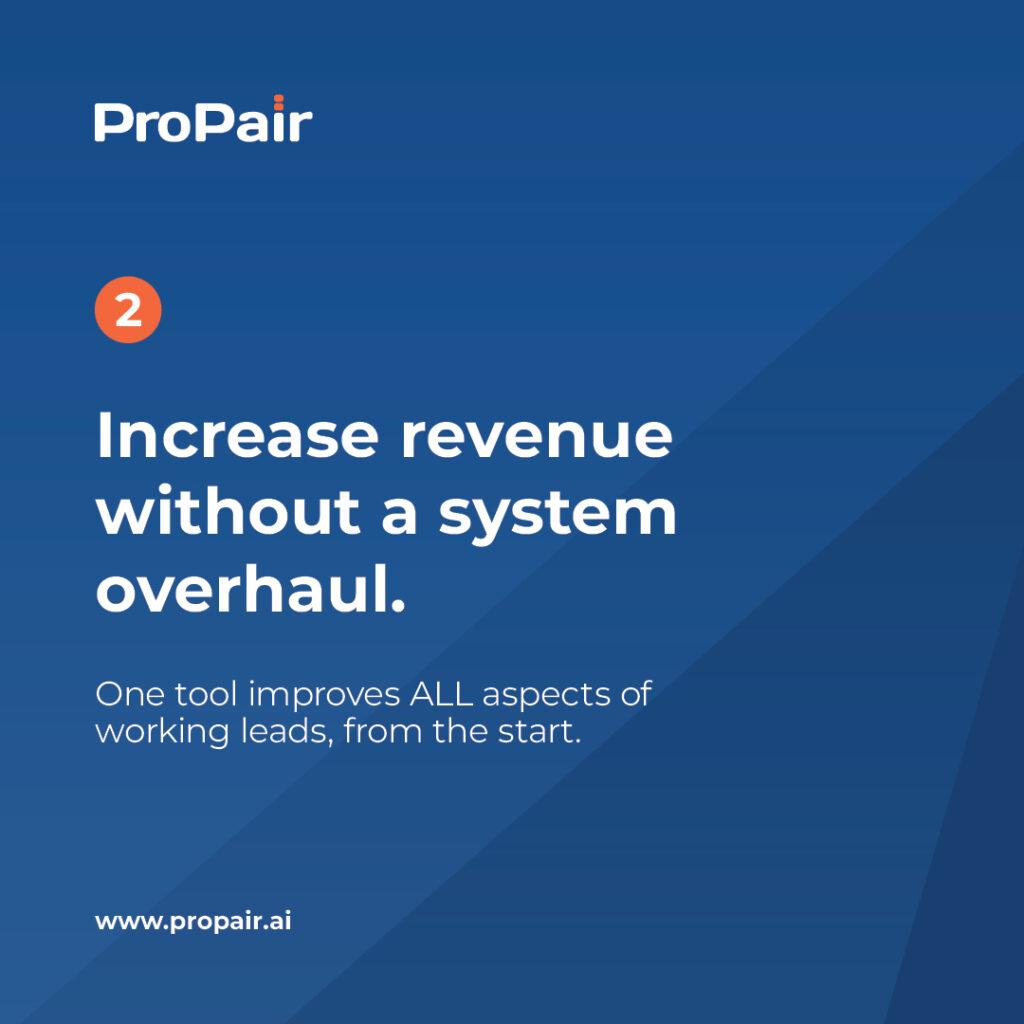
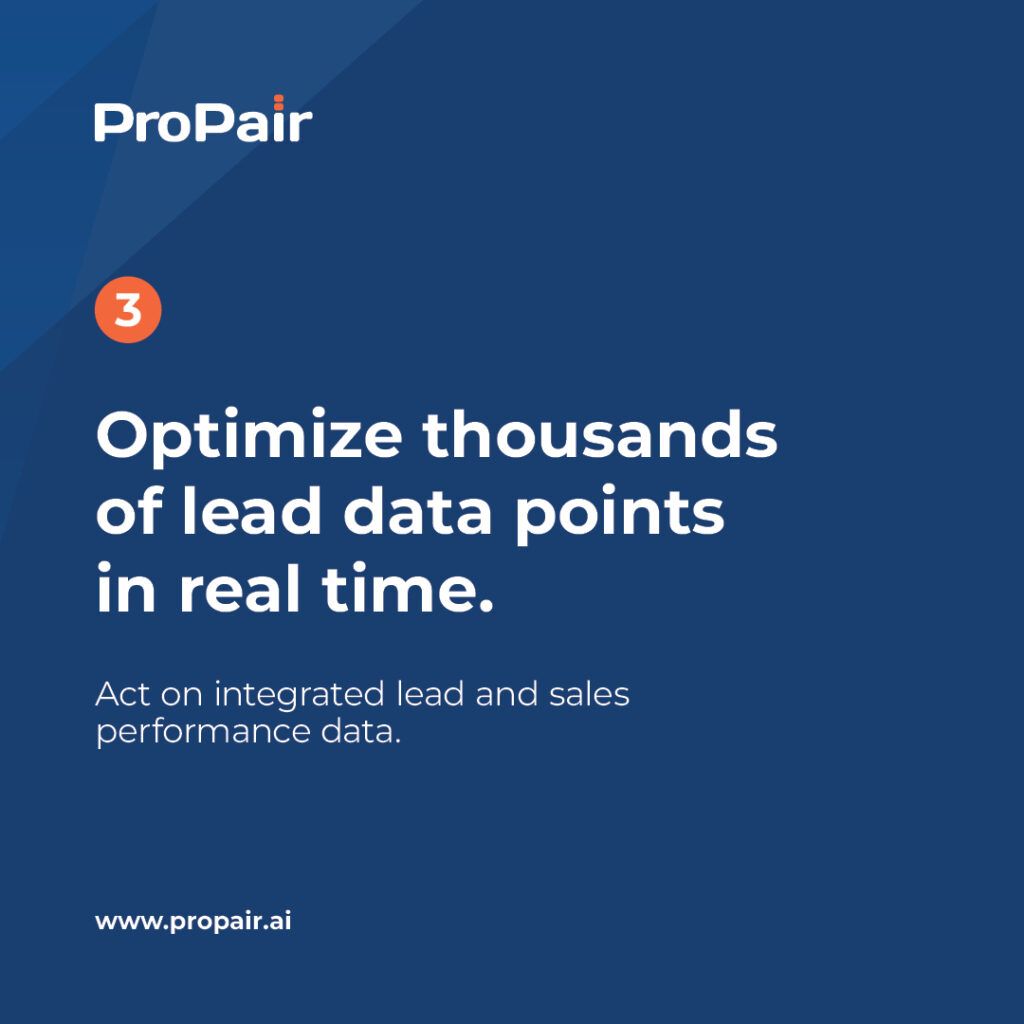
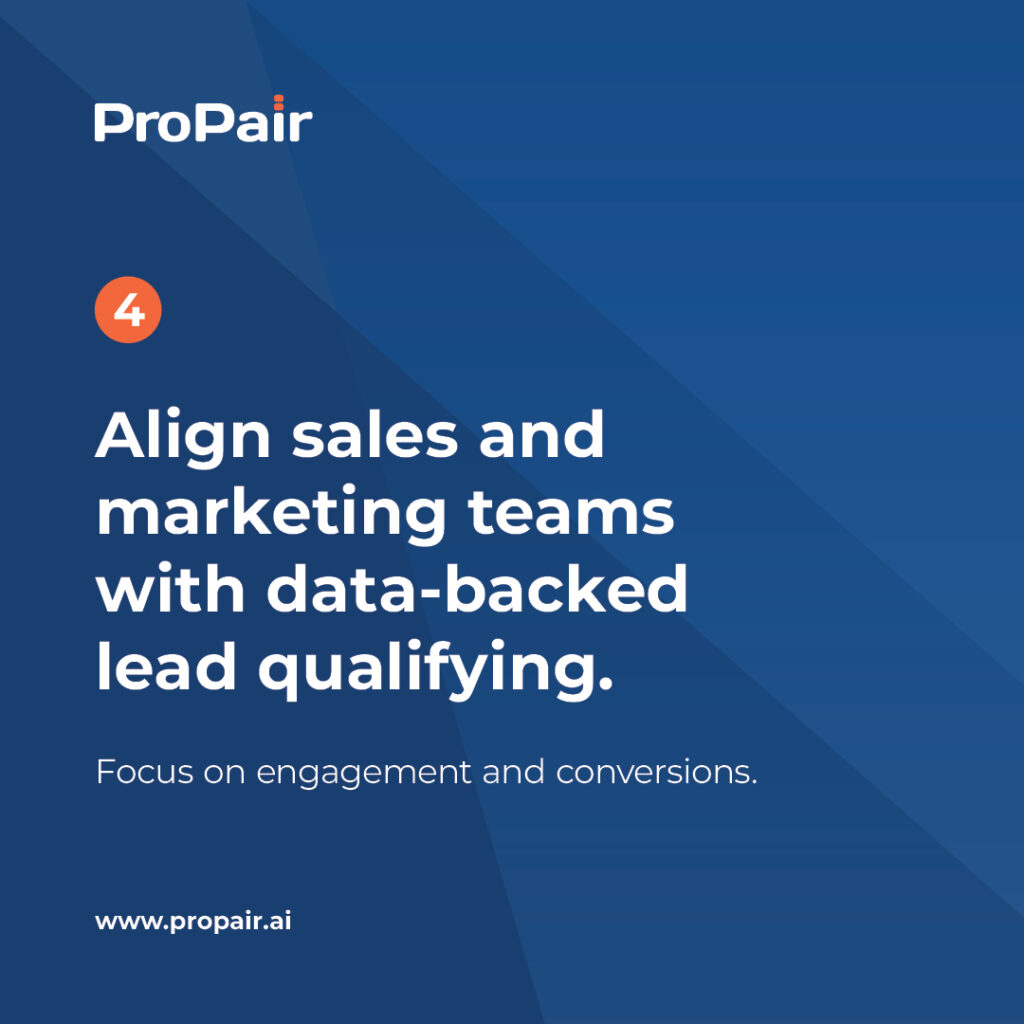
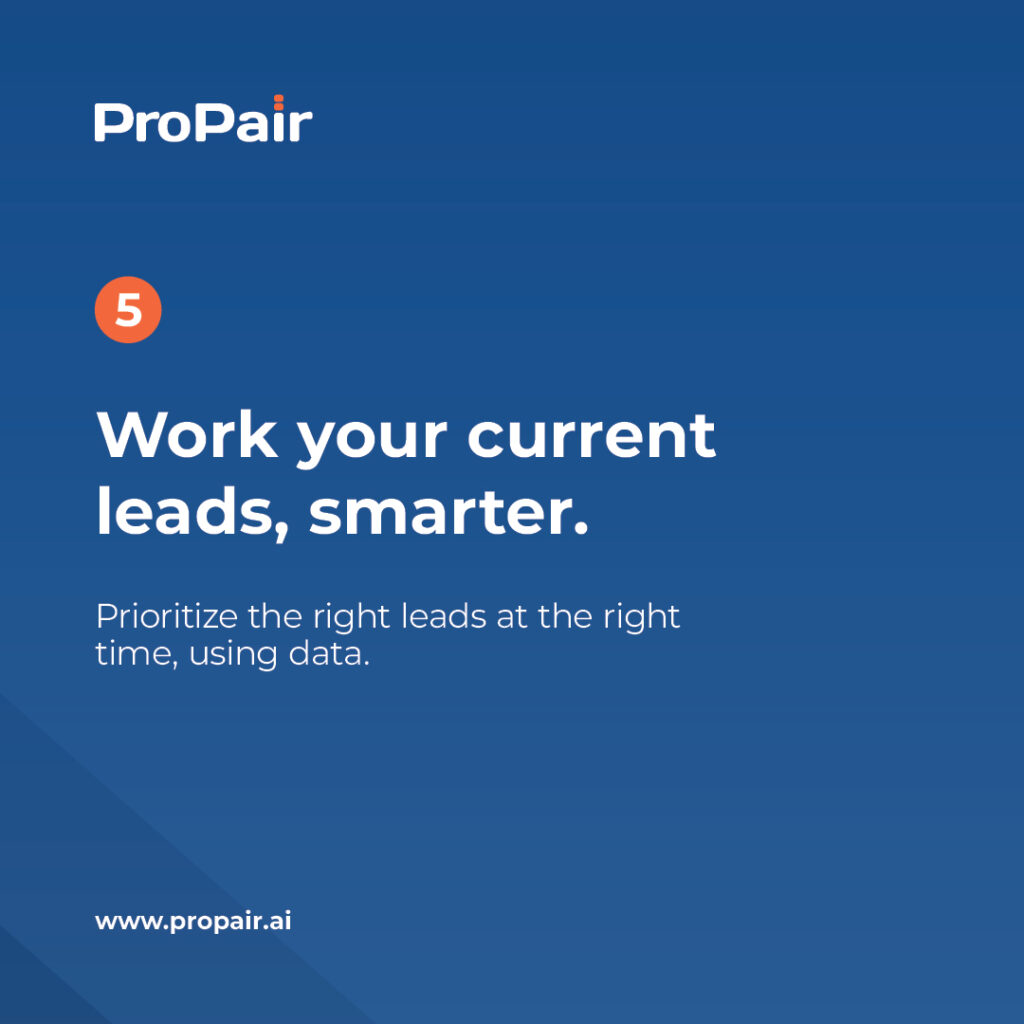
1. You waste leads without it
Without a reliable system for scoring leads, your sales team is likely making assumptions about leads that flow in.
Whether they think leads have aged out, aren’t qualified, or are just flat-out unresponsive, chances are, a deeper look at your lead data would reveal several missed sales opportunities because sales activities aren’t currently data-driven.
2. Increase revenue without a system overhaul
Leads flow in and the marketing and sales team do their best with the support they have. But what if your leads, marketing and sales teams could be optimized with one simple upgrade?
A high-quality lead scoring model increases efficiency, impacting all aspects of working leads, including what leads are generated or acquired, how they’re prioritized, and what sales actions are taken to convert them. When each of these steps is improved, ROI increases as your business closes more sales, more efficiently.
3. Optimize all your sales and lead data
You’re already collecting thousands of lead data points and you have access to performance data for your sales and marketing teams. But this data is overwhelming to manage.
A lead scoring model makes use of this data as leads flow in, prioritizing them, ranking them, and distributing the best leads to the right team members, at the right time.
4. Align sales and marketing teams using data
With data-driven lead scoring models, sales and marketing teams reach common ground based in fact, rather than assumptions, theories or static rules.
Lead scoring models rank leads, so there’s no more guessing as to what makes a qualified lead. Sales and marketing teams are empowered to move forward, doing what they each do best to engage and convert those leads.
5. Work your current leads, smarter
With a data-backed lead scoring model, your business can prioritize the right leads at the right time and engage them in the best ways possible.
Taking the guesswork out of this initial step in lead management launches the entire lead flow successfully. Customers and your sales and marketing team have an improved, more efficient and intelligent experience.
What is a lead scoring model and how do you establish one?
A lead scoring model is a structure that is used to establish and maintain a lead scoring strategy by providing the framework used to assess the value of each lead based on a variety of criteria.
With the best lead scoring models, organizations are able to quickly identify qualified leads and prioritize how to work them as they come into the organization’s CRM system.
How to use a lead scoring model to determine the value of leads
Within a lead scoring model, different criteria are established for assigning values or points to each lead to determine how qualified it is. These different criteria can clue sales teams into how ready-to-buy a lead may be.
This could include:
- Lead behavior: Assess how leads engage with you through website visits, downloads, forms filled, phone calls, emails opened, etc.
- Lead demographics: Assess who leads are by evaluating how qualified they are based on information including their location, job title, age, industry they work in, income, etc.
Beyond information from the leads, assigning value to leads could also be based on thresholds that are set to measure leads by. As we mentioned, this could include goals and metrics set by marketing and sales teams.
For example, the marketing team might launch a new campaign to attract leads for a specific product. Leads of certain demographics, showing certain behaviors within that campaign, may hit a point that the marketing and sales teams deem more ready-to-convert than other leads. Those leads would then be prioritized and contacted by salespeople.
Lead scoring models can measure and assign value to leads using different methods and criteria. Different lead scoring models will get you different results.
Each model relates to specific criteria chosen to reach certain sales and marketing objectives.
Learn more about lead scoring models: How to Develop a Lead Scoring Model, and Why it Matters
The challenge with traditional lead scoring models
There are various tools available to set the foundation for a lead scoring model.
For example, many lead scoring tools are built on the concept of assigning values based on various behaviors. With this, the goal is to prioritize the leads with higher scores or that meet other thresholds such as being sales or marketing qualified. This assumes that these values are what make a qualified lead.
Tools like this do a great job of helping sales teams make sense of lead data that flows into their systems. But how do we know the criteria they use to evaluate leads, the basis of the model, will best determine what makes a lead convert to a customer?
This is where predictive lead scoring improves these models and ensures that the data-driven criteria being measured are analyzed and acted on in the most accurate way possible, improving performance across the board.
Beyond tracking and assigning value to lead data, predictive lead scoring tools establish a lead scoring model based on criteria that are established using artificial intelligence.
Machine learning continuously learns and updates its models over time, making the criteria by which leads are scored more accurate than was ever possible before.
Get ahead of the competition. Read: Why AI Lead Scoring is Essential for Raising Conversion Rates.
Predictive lead scoring innovations
To help organizations not only make sense of their leads but actually optimize them, technology has advanced to offer innovative AI lead scoring and lead management tools.
Managing thousands of data points by hand has never been realistic. Although some tools have made it easier, nothing compares to the advances that have come with the application of artificial intelligence and machine learning.
See how AI can impact how you work your data. Try ProPair’s free data analysis.
Artificial intelligence and machine learning
When you hear about AI, you might associate the term with technology that mimics human behavior. Have you thought about how this could positively impact operations at your organization?
For businesses, AI software supports tasks like automation, engagement, and quick data analysis. All of these simplify many repeatable processes we use, making them more efficient than ever.
Machine learning is one of the many subsets of AI. It’s also one of the most practical applications of AI in business because it’s fairly straightforward in how it performs.
It uses algorithms that allow for input of data (like analysis of leads) and output of data (like predictions and decision-making to help you prioritize and take action with those leads).
So you can see how these would present an innovative solution for ongoing lead scoring needs.
Learn more with our Simple Guide to Optimizing AI/ML for Business Operations.
Keep things moving with lead management automations
You’ve also likely heard about, and may be using, various types of automations within your business that impact stages of the sales funnel.
This alone is a helpful evolution to manage repeatable sales and marketing tasks like data entry and activity logging. But it makes lead management even better with AI/ML applications.
With basic automations, as leads begin to engage, marketing teams automate steps like sending emails and tracking lead behaviors. And as leads become SQLs, sales teams automate scheduling calls, leaving voicemails, and sending emails to each of them.
AI/ML takes automation a step further, helping marketing and sales teams avoid inefficiencies and conflicts between the two teams.
With AI/ML software, sales and marketing teams get predictive insights to inform their decisions and next steps. As these predictions are based on the accuracy and intelligence of machine learning models, miscommunications, assumptions, and human error are removed from the equation.
Intelligent and predictive tools automatically score, distribute, prioritize, and communicate with leads as they flow in as MQLs and move to SQLs. And they do it with intelligent accuracy that isn’t possible when done manually.
Sales Automations backed by AI/ML software are changing the game for sales ops. Learn more: Automate Moving MQLs to Sales Qualified Leads with AI/ML Solutions.
5 features a lead scoring model should have
1. It’s predictive
A lead scoring model without predictive artificial intelligence and machine learning is a model built on assumptions, theories, and stagnant rules no matter how strategic you are with establishing it. AI lead scoring, however, relies on analyzing thousands of lead data points to provide lead scoring that is not only accurate but actually built on complex informed predictions of what makes a lead more or less ready to close a sale. And these predictions update in real time as lead data changes.
2. It measures implicit and explicit data
Your lead scoring model should be able to take all lead data you have and turn it into useful insights, this includes tracking and analyzing both lead behavior and facts about the lead. implicit data, and explicit data.
Implicit data is tied to behavior, showing engagement with your marketing and website, for example. Explicit data is information that leads provide or that is accessible information about the leads such as their income, job title, home value, etc.
3. It tracks and notifies as lead scores change
It’s not enough for a lead scoring model to evaluate a lead only when it first arrives in your system. High-quality lead scoring continues to track and measure your leads’ behaviors and data, providing you with ongoing information that informs whether a lead is becoming more or less sales-ready.
4. It supports the next step in the funnel
The value a lead scoring model assigns to each lead needs to be actionable. It needs to be clear to your sales and marketing teams what various lead ranks mean. From there, when a sales agent is notified of a change to a lead’s score, for example, they will be empowered to take their next sales action with that lead and keep them well prioritized with other prospects they’re working.
5. It benefits both marketing and sales objectives
As lead scoring involves tracking lead data that impacts both marketing and sales efforts, the lead scoring model needs to be reliable enough for both teams to trust. The more intelligent and data-driven the model is, the better informed sales and marketing will be. This also prevents any disagreements over deciding on certain thresholds or criteria used to measure leads, as it is all instead driven by concrete data.
Lead scoring tools
To optimize lead scoring in action there are many robust, production-ready lead scoring tools available.
They differ in various ways, including how they integrate with your CRM, what lead scoring model or models they use, how customizable the models are and whether lead scoring is predictive or not.
These different factors can impact which platform will help your sales team increase conversions. We’ve summarized a few of the popular tools to give you an idea of the options available.
Check out our Guide to Predictive Lead Scoring and Why Most Lead Scoring Models are NOT Predictive.
ProPair
ProPair’s AI/ML software offers predictive lead scoring with decision support through its products RANK, MATCH and MIX.
Not only does ProPair use machine learning to analyze your historic lead management data and immediately begin scoring leads, but it’s also customized to your current system, your goals and your business. This allows ProPair’s clients to lift conversion rates without changing anything in their current system.
How it works
ProPair runs in the background to analyze 30+ lead dimensions as leads come in, providing you with immediate predictive values, customized to provide strategic decision support.
With ProPair RANK you’ll see exactly which leads to focus on and when, using one data-driven lead value ranking system designed to help sales teams prioritize leads, guide follow-up activities, and revisit abandoned opportunities.
Bonus: A broader impact on overall lead management
In addition to lead scoring, ProPair also supports other aspects of lead management to increase conversions with AI/ML tools for sales agent scoring and lead distribution.
ProPair MATCH shows exactly what sales agents to assign leads to. This turnkey solution leverages historical sales team performance data and machine learning technology to equitably get the right leads to the right sales agents and make the most of your current team as it stands today.
ProPair MIX maximizes the potential of every lead and every sales agent, bringing together the best of predictive lead scoring and predictive sales agent grading.
It allows you to optimize your entire sales operation and equitably distribute leads from top to bottom performers, maximize sales production, and reduce the need and expense of churning your salesforce.
Get more from each lead with AI-powered lead nurturing. Download our free guide here.
Hubspot
Hubspot Marketing Hub offers predictive lead scoring software that works with your CRM to apply machine learning, which allows for reviewing thousands of data points across your contacts to identify your best leads.
How it works
Hubspot’s lead scoring model evaluates leads based on their behavior and engagement, demographic information, relationship within Hubspot and logged interactions within the CRM. From there it uses artificial intelligence to optimize lead scoring over time.
As you collect more data, Hubspot’s model improves itself providing better-informed predictions. It categorizes leads using “Likelihood to close,” which shows the probability of a contact closing within the next 90 days. The “Contact priority” feature uses “Likelihood to close” to filter segments of your best and worst leads.
To collect enough significant data to see Hubspot’s “Contact priority” assigned to leads, Hubspot says you’ll first need to reach 100 contacts.
Bonus: A wide range of lead scoring possibilities
Hubspot includes features like creating different score sheets for different audiences or leads for sales and marketing teams. It allows for up to 25 unique lead scoring models to help manage various products, locations, industries, etc. And there are options to customize how leads are scored to make it work for your team.
Marketo
Marketo Engage offers Lead Scoring Model as part of its marketing automation platform.
Its model categorizes users as leads, which have the potential to become customers, or prospects, which are customers who have engaged and showed interest in products.
How it works
With Marketo’s model, leads scored above 65 points are considered sales-ready. Leads below that point are either unqualified or may need to be engaged and analyzed differently to increase their lead score. This threshold can also be customized for your organization so sales and marketing will need to align on what that threshold should be.
Marketo combines explicit (demographics, basic lead information) and implicit (behavior and actions leads take to engage with your marketing) information to score leads. Note, this data needs to be kept up to date for accurate lead scoring.
Bonus: A focus on engagement to support sales and marketing
Marketo Engage’s focus is in the name — engagement. Beyond its Lead Scoring Model, Marketo Engage also makes it easy to track lead behavior in one central hub so you can optimize how you connect with leads. Push content by creating journeys that use the right channels, to reach leads at the right time, optimizing engagement.
Salesforce
Salesforce offers various ways to integrate lead scoring into your lead management. As a CRM it integrates with other tools like Hubspot and Marketo to take full advantage of their lead scoring capabilities within Salesforce.
There are also extensive options to customize your use of Salesforce using workflow rules to establish lead scoring methods within the platform. Because this takes time to develop and advanced functionality to execute well, Salesforce also offers a more ready-made solution for lead scoring — formerly Pardot, now called Marketing Cloud Account Engagement.
Using Account Engagement, it’s easy to attach lead scoring actions and data to each lead within Salesforce. In addition, Salesforce offers Einstein to take lead scoring further by making it predictive.
How Account Engagement (formerly Pardot) works
Account Engagement is a marketing automation platform that allows you to define the value or weight of various lead data and actions within the sales funnel.
A lead scoring model is built based on actions leads take on your website and through your email campaigns.
Based on chosen values, when a lead reaches a certain threshold, sales and marketing teams then define the lead as qualified. Once a lead is deemed qualified, the sales team can prioritize working the lead.
Account Engagement’s lead grading system compares how well each lead matches to your Ideal Customer Profile (ICP). It automatically analyzes lead’s basic data to assign a letter grade of A through F to each lead.
To determine if a lead has a high grade, and is, therefore, worth prioritizing, it assesses demographic information, such as the lead’s location, revenue, job title, industry and company size. In addition, it can also track technographics, including CRM usage, marketing automations, etc.
Account Engagement (again formerly Pardot) also makes it possible to assign value to various actions, including web page visits, links clicked, downloads, email open rates and clicks, searches and other engagement with marketing campaigns and materials.
Bonus: Einstein adds predictive lead scoring
To take lead scoring further, Salesforce offers Einstein as an add-on to offer a predictive lead scoring approach.
Using existing lead data, Einstein’s AI software finds data points that have pointed to a lead’s successful conversion. With this information, it automatically shapes the model that leads should be scored by. The higher it scores a lead, the more likely that lead is to convert.
Einstein scores each lead by using a correlation between new lead attributes and those of historical leads.
It offers tools including Discovery to show relevant patterns in your data and AI insights, Prediction Builder to predict business outcomes and create custom AI models using fields or objects, and Next Best Action to deliver recommendations and action strategies.
Ready to start increasing sales? Check out our Guide to Implementing AI/ML for Executives in Sales Operations.
Lead scoring examples and use cases
Lead scoring is used across industries to improve the process of selling a product or service.
Let’s dig into a few real-world examples of how lead scoring can be tailored to improve marketing and sales operations within industries that benefit most from quality lead scoring.
The universal basics that apply across industries
As we’ve mentioned, lead scoring involves collecting both implicit and explicit data on leads, from tracking their basic demographics to their behaviors in engaging with your organization.
Across industries, there are various data points you can collect to improve how you score and manage leads once they’re generated. This is often done well through a lead-capture form on your website, or other marketing tools, that asks leads to provide information about themselves.
In addition to this, lead scoring can be supported by pushing targeted marketing campaigns to attract and nurture leads, which you can use to measure their behavior as they engage with the materials.
All that said, depending on the lead sources and lead scoring tools you use, you may not need to stress about how you’ve structured collecting lead data through these tactics, as the tools and sources might offer helpful support.
Make the most of the leads you already have. Download our free guide to maximize aged leads with AI.
Mortgage lead scoring
Mortgage lenders will want to collect data that specifically helps loan officers gauge whether a lead is ready to close a loan. This includes data like credit score, income, debt amount, home value, etc. These data points should also be considered in relation to several different loan programs the lender offers.
One aspect of scoring these leads and deciding how to prioritize and work them may be to distribute them to the loan officers who connect best with leads in that location or with certain levels of credit score, for example.
Beyond the lead data, lenders may also want to provide marketing materials and campaigns that meet a lead where they are in the process of considering a home loan and help to move them along the path to be ready to close. This could include blogs and emails aimed at educating homebuyers and homeowners about their financing options.
With intelligent, predictive lead scoring, all of these various factors — between leads, sales agents and relevant loan products — can be combined using machine learning. Rather than assess one or two dimensions, many complex dimensions can be summarized into simple predictive values that help lenders prioritize and work each lead.
Read More: Perfect Your Mortgage Lead Scoring and Sales Process with Intelligence Technology.
Financial services lead scoring
Beyond mortgage lenders specifically, various financial services organizations benefit from effective lead scoring. This includes banks and lenders providing student loans, auto loans, other personal loans, business loans and debt consolidation.
In this case, qualifying a lead may also be based on their credit score, as well as income, debt, financial needs/goals, and where they are in the process of looking for a financing solution.
All of these points allow you to prioritize a lead based on how ready they are to move forward with the solution you have to offer.
Leads can be attracted and nurtured through various marketing efforts that show the benefits of your loans compared to other options, as well as how the financing can be simple and affordable.
Predictive lead scoring provides decision support to help you know what sales activities to take with each lead depending on the above factors.
Insurance lead scoring
Whether you’re selling health insurance, Medicare, life insurance, auto insurance or any other form of insurance, you have certain criteria that leads need to meet before they’re ready to get started.
Depending on the type of insurance you offer, this could include collecting specific data such as a lead’s current state of health, their budget for insurance premiums, their current policies, if they’re the decision maker, etc.
As for helping to attract and move leads through the sales funnel, educational resources that build trust and show the need for the insurance you offer can be helpful to advance their decision to commit to your offering.
Predictive lead scoring models help you get familiar with each lead as they flow in, using the many dimensions needed, customized to your business.
How to make your lead data work for you
Depending on how hands-on you want to be and other considerations of your current lead management system, there is likely a lead scoring tool available that could help you sustainably prioritize which leads are sales-ready.
The beauty of tools based specifically in AI/ML capabilities is that lead scoring becomes completely automated while also being continuously updated so that the automations are also intelligent and more accurate than a manual system could ever be.
With machine learning, lead scoring relies on the accuracy of data and the machine’s ability to analyze and learn from thousands of data points in an instant.
With an option like ProPair specifically, your historic data and newly generated lead data don’t have to be perfect for you to see results. We help you adjust our model to best fit the needs of your organization overall, your sales team and your leads.
With our customized solution, you apply predictive lead scoring to your organization’s unique sales processes, lead buying, culture, and sales agents.
AI/ML lead management support can take what your organization already has in place to transform your efforts into creating the best version of you.
The top three most significant challenges companies face when considering the implementation of AI are staff skills (56%), the fear of the unknown (42%) and finding a starting point (26%). ProPair eliminates these concerns with its turnkey solution that runs in the background of your current system to support your sales and marketing teams.
Optimizing sales has a direct impact on revenue. Learn more in our Guide to Optimizing Your Revenue Operations.
Lead Scoring FAQ
What is lead scoring?
Lead scoring is the system that sales and marketing teams use to assign value to each new prospect, which is meant to determine how important the lead, or potential customer, is to the business. By ranking each lead based on various data points, sales and marketing gain clarity on which leads to engage and when, prioritizing leads based on the probability of each one turning into a sale.
What is a lead scoring model?
A lead scoring model is a structure that is used to establish and maintain a lead scoring strategy by providing the framework used to assess the value of each lead based on a variety of criteria.
Why should I use lead scoring?
When you invest in buying or generating leads, you pay for sales opportunities and receive thousands of rich data points. However, if lead data is not evaluated, and instead left static, it underperforms.
Lead scoring tools help you avoid the pitfalls of managing this data. Especially now, with complex models available that measure thousands of data points and combine them into one predictive lead scoring value, such as predictive lead scoring with artificial intelligence.
Does lead scoring improve sales?
Having leads is only valuable to your business if you can convert many of those leads into customers. Accurate lead scoring is one of the first steps to optimizing sales conversions as leads flow into your lead management system.
Lead scoring helps marketing and sales teams quantify how qualified leads are. This informs how to prioritize, distribute and work each lead to focus on sales-ready leads that increase conversions.
What criteria are used for lead scoring?
Lead scoring models use both implicit and explicit lead data to measure the probability of a sale.
Implicit data includes lead behavior such as assessing how leads engage with you through website visits, downloads, forms filled, phone calls, emails opened, etc.
Explicit data includes lead demographics. This involves assessing who leads are by evaluating how qualified they are based on information including their location, job title, age, industry they work in, income, etc.
What score makes a lead qualified?
This depends on many factors relevant to what makes a lead most likely to close a sale with your business. Scores are often weighted based on thresholds your marketing and sales teams agree to, such as what makes a sales or marketing qualified lead. These thresholds could be determined with help from predictive lead scoring tools that make sense of complex lead data.
What are the challenges of implementing lead scoring?
One big complication is relying on a lead scoring model or models that don’t serve your ongoing needs. A high-quality lead scoring system needs to measure leads readiness to buy as soon as they flow in, but also continue to measure how qualified leads are to make a sale as their behavior and demographics change over time.
This means you also need a system for ongoing tracking of lead data and a way to be alerted and take action once a lead becomes more or less valuable. With the right lead scoring system, you can wrangle complex lead data and optimize how you prioritize and work leads based on their scores, without having to manually update the model.
What are the limitations of lead scoring models?
Lead scoring models at their most basic are only as good as the data being fed into them and the criteria set for measuring that data. Using lead scoring models can be difficult when large amounts of data are managed manually and criteria are set based on assumptions or theories.
The limitations are fading however, with more intelligent models. Now through various tools, including predictive lead scoring, both data analysis and criteria measuring are done using algorithms. Artificial intelligence has transformed lead scoring into a data-driven tool that uses machine learning to continuously improve the predictive values used to score leads.
Boost ROI with strategic and intelligent lead scoring
Before reading this ultimate guide, you might have thought that lead scoring was one small part of your overall lead management system.
However, we hope you’ve come to understand that qualifying leads is actually the first step that impacts how you make use of the leads you’ve spent time and resources to generate or buy, as well as how you move those leads through to become customers.
With effective lead scoring and using the right lead management tools for your organization, you can boost lead scoring ROI and increase conversion rates.
How could your business grow with intelligent lead scoring?
Schedule your ProPair demo now to learn more.
Using machine learning to analyze your current leads and their performance, we’ll help you see where you’re missing sales opportunities.
We’ll also share the options you have for improving lead scoring, sales agent performance and overall conversion rates with our production-ready machine learning software.
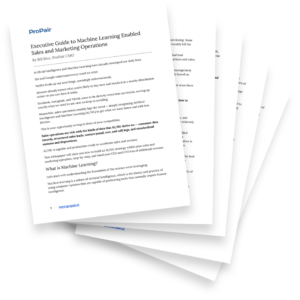
How does AI increase sales?
Download our executive guide to understand the current state of AI and machine learning. We’ll show you how innovative sales and marketing organizations use it to get ahead of their competition.